Multicenter Data Streaming for ML-Development
See all Use Cases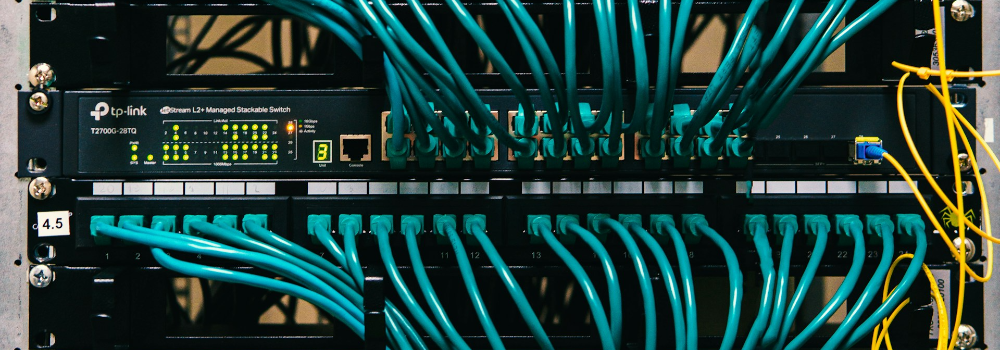
Multicenter Data Streaming for AI-Driven ML Development
Overview
A collaborative network of university hospitals treating Parkinson’s disease and other movement disorders faced the challenge of integrating diverse clinical data from multiple sites. By adopting dotbase, they built a central, real-time platform that harmonizes routine-care records, unifies workflows, and stores all information in one place. This paved the way for accelerated AI research in Deep Brain Stimulation (DBS).
Partners
Multiple university clinics and specialized movement-disorder centers
Setting
Academic hospitals with varied documentation standards and legacy IT systems; transregional research network
Challenges
- Fragmented Data: DBS patient records were dispersed across incompatible databases, impeding cross-center analysis and slowing treatment improvements.
- Inconsistent Documentation: Non-standardized clinical notes made it difficult to compare outcomes and track patient progress.
- Time-Consuming Workflows: Researchers spent countless hours manually extracting and cleaning data before any AI model could be trained.
- Siloed Collaboration: Neurologists and data scientists operated in isolated workflows, leading to significant data breaks and miscommunication.
Our Solution
Implementing dotbase delivered a unified, AI-ready infrastructure across the entire hospital network:
- Standardized Clinical Templates
Uniform templates for capturing DBS procedures, patient responses and follow-up metrics. - Cross-Site Data Harmonization & Centralized Data Repository
All harmonized records reside in one secure platform, ensuring data integrity and easy access. - Real-Time Research Interface
Interactive dashboards where clinicians can tag, flag, and annotate cases, directly feeding ML pipelines. - Collaborative Workflows
Shared, role-based views enable neurologists, surgeons and researchers to work together seamlessly.
Outcomes
- AI-Ready Datasets: Consistently labeled, high-quality data now powers faster machine learning experiments.
- Time Savings: Dramatic reduction in data-preparation effort, freeing up researchers to focus on model development.
- Enhanced Clinical Decisions: AI-supported decision trees can guide more precise DBS programming, improving patient outcomes.
- Scalable Architecture: Easily extendable to other neuromodulation therapies and additional hospital sites.
Conclusion
By unifying their data through a networked implementation of dotbase, several university hospitals have transformed care and research into a seamless, AI-centric ecosystem. Clinicians and scientists now collaborate in real time in a harmonized workflow and centralized data storage to drive faster discoveries and personalized neuromodulation therapies.